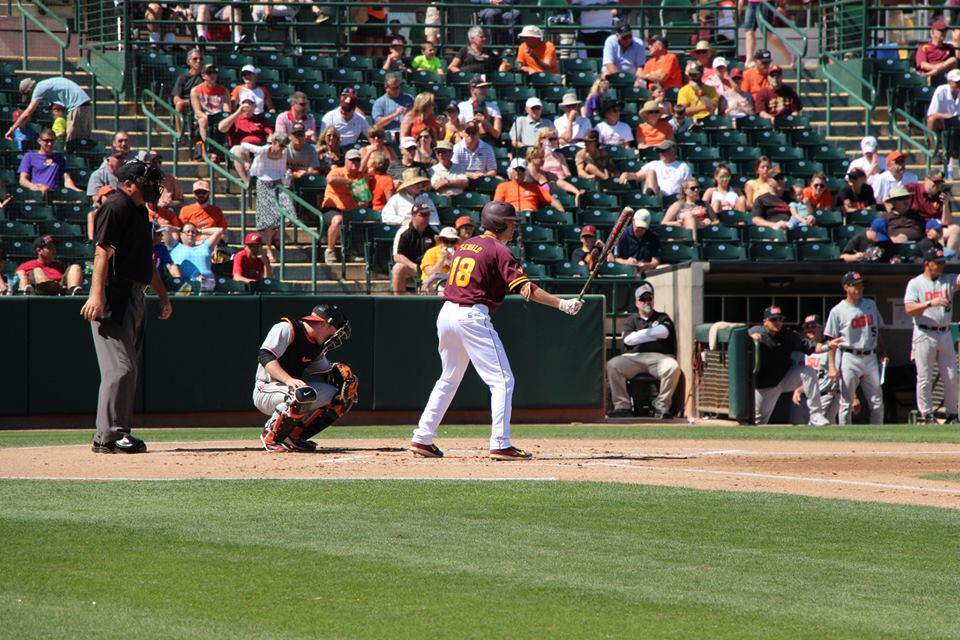
(Photo: Dominic Cotroneo/WCSN)
For as rapidly as sabermetric stats have infiltrated Major League Baseball, the college baseball landscape has gone largely untouched.
A number of factors go into why this is the case, ranging from the absence of expensive data tracking technology to a general satisfaction with the status quo.
Still, that’s not going to stop us from having some fun.
What follows is a primer of three sabermetric stats and an analysis of the Arizona State offense from a sabermetric standpoint. Because the complexity of available stats is somewhat limited and I thus don’t have access to line drive, ground ball or fly ball rates, park factors or League Average wOBA’s (weighted on base average), some of the more fun advanced stats like WAR (Wins Above Replacement), hard-hit rate, wRC (weighted runs created) and UBR (Ultimate Base Running) have to be left out.
Instead, this article will focus on the interesting findings that my by-hand calculations of ISO (isolated power), BABIP (batting average of balls in play) and wOBA yielded for individual ASU players.
The pitching version of this article will be released after the Sun Devil’s weekend series against Stanford.
Isolated Power (ISO)
Calculated by the equation Slugging Percentage-Batting Average, ISO measures a player’s raw power by yielding the rate at which the player hits for extra bases. From a conceptual standpoint, ISO and slugging percentage are quite similar. But from a practical standpoint, ISO is much easier to process, given how slugging percentage often needs to be paired with batting average to get an accurate assessment of a hitter.
Courtesy of FanGraphs, here is a table that details what is desirable and what is not-so-desirable at the Major League Level.
Rating | ISO |
Excellent | 0.250 |
Great | 0.200 |
Above Average | 0.170 |
Average | 0.140 |
Below Average | 0.120 |
Poor | 0.100 |
Awful | 0.080 |
Applying ISO to ASU’s most notable hitters, the rankings are as follows:
- Dalton Dinatale: .244
- RJ Ybarra: .191
- Trever Allen: .150
- Colby Woodmansee: .108
- Brian Serven: .096
- David Greer: .089
- Jordan Aboites: .086
- Jake Peevyhouse: .085
- Joey Bielek: .048
- Johnny Sewald: .000
Again, the above table is an indicator of what a certain rate looks like at the professional level. Given the depressed run-scoring environment in college baseball, it’s not shocking that over half the ISO’s for ASU’s offense are uninspiring—the same would be true for any other college team.
Still, there’s much to learn from these findings.
Perhaps most importantly is the fact that Dalton Dinatale has evolved as a hitter and absolutely merits a spot in ASU’s lineup when his fractured thumb heals. His sample size may be quite a bit lower than that of Ybarra, Allen and Woodmansee, but his knack for extra bases this season was apparent from the start.
On a less positive note, Johnny Sewald has yet to record an extra base hit this season, and his ISO has yet to lift off the ground as a result.
Batting Average on Balls in Play (BABIP)
The naysayers may claim that recovering from a fractured thumb could zap Dinatale of his early-season power and thus cause his ISO to plummet.
I say, one look at his BABIP before the injury, and there’s no reason to assume that he won’t be even better upon return.
First though, let’s introduce the concept of BABIP.
The acronym sounds intimidating, but rest assured, it’s one of the most useful baseball stats to have in your arsenal.
Calculated by the equation (H-HR)/(AB-K-HR+SF), BABIP tells us the rate at which a batter gets a hit when he puts the ball in play—essentially, a player’s luck factor.
FanGraphs gives a lengthy explanation of the ins and outs of BABIP here, but I’ll try and boil it down in a more concise manner.
When considering BABIP, think about the paradoxes of baseball – hitting in particular. A ball that is crushed, but right at a defender, is going to look the same in the box score as an infield popout. A soft Texas-leaguer that falls in for a base hit is going to look the exact same as a screamer up the middle.
BABIP accounts for this luck factor.
The MLB average BABIP is .300, meaning that most players gravitate towards this mark as the season wears on. Simply put, if a player’s BABIP is exceptionally high, resting around .400, it’s safe to assume that the production is fueled at least in part by luck and the player is primed for regression. Conversely, if a player’s BABIP is much less than .300, unluckiness has been playing a factor and the player’s numbers will see an uptick once more balls in play start falling in for base hits.
With that in mind, here are the current BABIP’s of the same list of ten hitters that was showcased above. The order is arranged this time in terms of ASU’s normally constructed lineup card:
- Johnny Sewald: .324
- Jake Peevyhouse: .375
- Colby Woodmansee: .384
- RJ Ybarra: .316
- Trever Allen: .408
- Brian Serven: .380
- Joey Bielek: .375
- David Greer: .389
- Jordan Aboites: .236
- Dalton Dinatale: .257
Once these results have been processed, consider the caveat about BABIP: It’s also heavily influenced by quality of contact. That is, a player is more likely to get a base hit on a ball that is hit hard, and less likely to get a base hit on a ball that is hit softly. In terms of BABIP, the harder the contact, the higher the BABIP.
Since collegiate baseball doesn’t have contact-tracking technology, this is where ISO comes into play. Fears of a dangerously high BABIP (for example, Woodmansee’s .384 mark and Allen’s .408 mark) should be dispelled because of the backing of a high ISO. Because these two players make harder contact more often than many of their teammates, their higher BABIPs are sustainable.
The same cannot be said for a player with a low ISO and a high BABIP.
Apply that logic to Dalton Dinatale, and that’s why I expect him to rake even more when he comes back. He has a high ISO, which means he routinely hits the ball hard, AND his low BABIP implies that he was actually slightly unlucky before his injury.
Weighted On Base Average (wOBA)
Saving the best for last, wOBA is an ultimate hitting stat.
Sabermetricians are united under the noble idea that scoring runs is the most important aspect of hitting, and the advanced stat of wOBA reflects this assertion.
The formula–
(0.690 x BB) + (0.722 x HBP) + (0.888 x 1B) + (1.271 x 2B) + (1.616 x 3B) + (2.101 x HR)
AB+BB+SF+HBP
–is complicated.
At the same time, the idea behind it isn’t difficult.
Each possible positive outcome in an at-bat is factored into the above equation and each is assigned a weight (the number in front of each outcome) based on how much the outcome contributes to producing a run.
Combined, batting average, slugging percentage and OPS essentially mean the same thing as wOBA does. But wOBA reigns supreme, as it’s more accurate in the weights it places on individual outcomes and it’s a single stat doing the power of three.
Here’s a table from FanGraphs that details acceptable rates for Major Leaguers. Notice how it is in sync with acceptable on-base percentages:
Rating | wOBA |
Excellent | .400 |
Great | .370 |
Above Average | .340 |
Average | .320 |
Below Average | .310 |
Poor | .300 |
Awful | .290 |
Accordingly, here are the top ten hitters in the Sun Devil offense, based on wOBA:
- Trever Allen: .392
- RJ Ybarra: .383
- Dalton Dinatale: .378
- Brian Serven: .373
- Colby Woodmansee: .367
- Jake Peevyhouse: .363
- David Greer: .361
- Joey Bielek: .346
- Johnny Sewald: .309
- Jordan Aboites: .254
Where wOBA falls short is that it stops measuring production once the hitter leaves the batter’s box. In other words, leadoff hitter Johnny Sewald looks like a mediocre offensive contributor based on his .309 wOBA. What wOBA doesn’t account for is Sewald’s speed and his ability to put himself into scoring position without necessarily hitting for extra bases. Thus, he’s more valuable to the offensive engine than his wOBA implies.
It’s worth noting that sabermetric stats, especially stats like wOBA and ISO, aren’t designed to replace traditional stats like batting average, RBI and slugging percentage. Rather, balancing the two allows for both sets of numbers to complement the other.
Using these stats creates more tools in the toolbox, allows for more informed predictions about future success and is ultimately more telling of a player’s overall ability.
Follow Jacob Garcia on Twitter @Jake_M_Garcia or connect with him on LinkedIn.